How Data Tokenization Affords Analytical Value
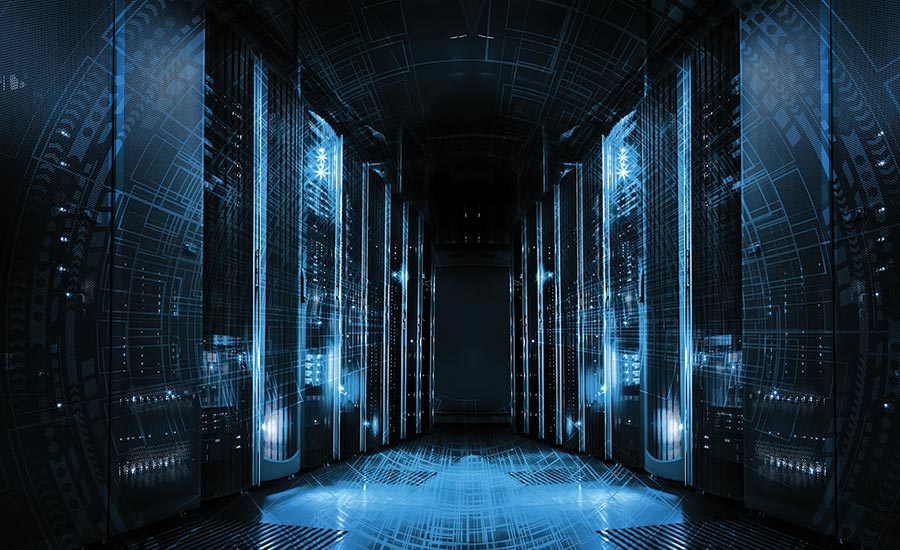
Regulations like CCPA and GDPR hold companies that process personally identifiable information (PII) accountable to a minimum standard of data protection. This means that enterprises are expected to prevent unauthorized access to private information while simultaneously securing perimeters from potential external threat. With the rise of data analytics, compliance is becoming an increasingly difficult challenge, particularly as the majority of protected information being processed by private enterprises and governments is stored in both disparate and dissimilar databases on premises and in cloud resources. As part of an effective analytics framework, data amalgamation is typically required to gain the best insights and outcomes – which poses a significant challenge to also maintain regulatory compliance and is further complicated by variations across geographical borders. In a best-case scenario, these challenges create an awkward situation and in a worst-case scenario, can lead to vulnerable data or unauthorized access with an increasing number of attack vectors.
As data security regulation varies from country to continent, so too does its interpretation. There have been many legal debates about the definition of privacy provisions written by legislative bodies globally. Uncertainty breeds confusion, and many organizations are falling through the gaps left by regulatory frameworks due to budgetary challenges and various uncertainties.
Preventing Analysis
The best way for companies of any size to protect sensitive data is to ensure that security is built in as standard. While it can be difficult for established organizations to retrospectively integrate security standards, it is essential endeavor. Unfortunately, many boards will prioritize operations over complete security, thereby putting critical systems at risk. However, what they don’t account for is the very real threat of breached data or regulatory fines nor the risk to reputation as long-term fallout. Data shows that in the long run it is much more cost effective to deploy data protection before it is needed.
The best way to ensure that data is completely secured is to deploy a data-centric strategy that focuses on securing the data itself instead of simply putting up walls around the information. This means more than limiting access to data on a need to know basis because this also encumbers sharing data and analytics. When it comes to data analysis, it is objectively best to have full fidelity of a dataset to ensure that the full picture is visible. Many of the current data privacy standards stipulate that data cannot be shared unless is has been anonymized or pseudonymized which can makes it almost impossible to extract meaningful value from collaborative data – akin to converting a full color photograph, rich with shading and nuance, to a two-tone black and white photograph devoid of any enjoyment. The regulatory motivations are well intended since it is logical that the fewer people that can access and read sensitive information, the more secure the information is. However, this makes it difficult when enterprises want to combine their insight with others, as doing so may put them at risk of breaking compliance laws.
The Solution
Recently, data tokenization has proved to be a successful protection method for securing sensitive information and all instances of personal data. This is because it allows information to retain its analytical value, while ensuring that it meets regulatory requirements. Tokenization replaces sensitive data with tokens that maintain the analytic value of the original data without compromising its security or running awry of privacy concerns. It allows for the preserving of the characteristics of the data such as the type (numeric, alpha, alphanumeric) and length which makes implementation easier because some systems are sensitive to data type and length. Unlike traditional encryption, which renders the data analytically inert without decryption, tokenization retains analytic value and fosters the ability to use data in its protected state. Tokenized datasets still have full referential integrity and the same statistical distribution as the original data.
What allows data tokenization to stand out against other anonymity-providing techniques is its unique ability to facilitate sharing data, in its tokenized form, without compromising security by revealing or sharing sensitive data. This allows information to be analyzed and utilized without being askew of regulatory framework, which can result in both loss of trust and hefty fines.
The Application
Tokenized data intrinsically opens up analytic possibilities in nearly every vertical. Because tokenized data is typically defined as "pseudonymised" data, it cannot be attributed to a specific data subject. While this doesn’t make one exempt from regulatory compliance, it is certainly a step in the right direction. It is interesting to note that, in the context of the CCPA, information is not “personal information” if it has been “deidentified.” This means that tokenized data is accepted as a form of compliance under CCPA. This is huge from a CCPA compliance cost reduction perspective as pseudonymized information might not be considered “in scope” for CCPA audits.
Likewise, in the healthcare sector, where information is also subject to additional regulation, such as the previously mentioned CCPA, and Health Insurance Portability and Accountability Act (HIPAA), which stipulates how PII or PHI (personal health information) should be protected. According to HIPAA, “there are restrictions on the use or disclosure of de-identified health information. De-identified health information neither identifies nor provides a reasonable basis to identify an individual.” This means that tokenized information enables regulatory compliance for both HIPAA and CCPA, while facilitating the sharing of sensitive information between agencies without compromising security. This is especially critical to analytics in this sector which are often based on longitudinal studies and the need for reliable referential integrity is a must.
As tokenized information opens up a whole new realm of possibilities, we could soon begin to see its positive effects. For example, it could be used to track correlation between sensitive topics such as addiction or serious illness by examining habits, age and location all without revealing any PII or PHI. If used properly, tokenized data may even be able to help tackle the opioid crisis in North America. Furthermore, with the sudden rise in the need to perform contact tracing as a critical response to the COVID-19 pandemic, tokenization can act as a true enabler – secure data collection, secure data storage and secure data analysis – a de facto trifecta where privacy, compliance and outcomes that save lives can come together.
By tokenizing the personal aspect of data such as PII and PHI, agencies can collaborate more effectively without compromising privacy, and addressing regulatory concerns by de-identifying information. This means that corporations will be able to extract more meaningful insight from sensitive data while simultaneously reducing the risk of data abuse by third parties. This means that even if third-party security practices are substandard, the security of the information will not be compromised – thereby improving the security posture and increasing collaborative potential.
Looking for a reprint of this article?
From high-res PDFs to custom plaques, order your copy today!