Big Data in Motion – Real Time Analytic Solutions for 21st Century Challenges
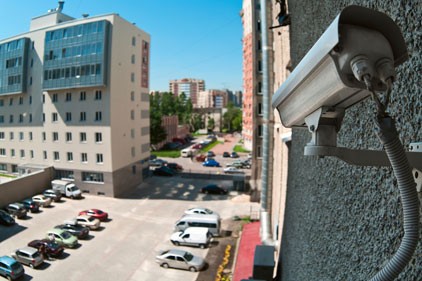
In the decade since 9/11, the United States has invested enormous resources into protecting our critical infrastructure from asymmetrical attacks, such as car bombs and hijacked airplanes. The problem is that our most vital facilities – pipelines, ports, refineries and power plants – are also vulnerable and difficult to secure due to their remote locations. More daunting is the fact that most of these facilities utilize Web-based command, control and communications technology that leaves them open to cyber-attacks.
We have responded to physical threats in two ways. First, by hardening these facilities whenever possible, and secondly by installing layers of sensors that warn of approaching danger. A single facility may be wired with listening devices, motion detectors, video cameras, magnetometers and a litany of other sensors that attempt to identify and pinpoint security threats. Although less widely deployed, network flow sensors have also been developed to detect cyber incursions into our networks, which represent a cooperative blend of U.S. Government monitoring and the private sector investments.
The challenge, of course, is monitoring the huge volume of data flowing in from these networked sensors. By now, everyone has heard of the challenges of Big Data. But for security professionals involved in infrastructure protection, the real problem is dealing with Big Data in Motion. If we collect the incoming sensor data and wait for two days or even two minutes to analyze it, that’s probably too late to stop an attack. Big Data has to be analyzed as it’s flowing in at high velocity from the sensors, not afterwards when the data is static. Data at rest has less value.
Big Data in Motion, therefore, presents processing and analysis challenges related to data Volume and Velocity, as well as a third component – Variety.
Security professionals, especially in the defense/intelligence community, have found that threats can be identified earlier – and often predicted – through the integrated analysis of multiple sensor data streams in motion, while sometimes also comparing the dynamic new data against historical data at rest in a database. Data variety compounds the difficulties of processing because so many different types of raw data must be analyzed, often taking into account non-linear relationships between and among the data sets. And this has to be accomplished in as close to real time as possible.
The importance of analyzing multiple streams of sensor data in a holistic way cannot be emphasized enough. One anomalous situation, such as a strange vehicle caught on video in the parking lot, might not trigger concern. But if that incident coincides with an individual swiping their card key at a side door outside of normal work hours, it may be first sign of trouble. Without integrated analysis of these cyber and physical data sets – a technique known as correlative analysis – prioritizing the level of threat and appropriate response is more difficult.
The solution to the Big Data in Motion challenge lies in a rapidly emerging technology concept called Real Time Analytical Processing, or RTAP. We already see the fundamentals of RTAP at work in the models that continuously analyze stock market feeds, meteorological data, crop condition and even Twitter activity, all in an attempt to predict what’s going to happen next, whether one year or a split second from now. These models are constantly calculating and re-calculating the risk of specific events occurring until a pre-determined threshold is exceeded and an appropriate response is triggered.
The biggest names in Information Technology are focused on RTAP, and while much has been accomplished, many breakthroughs must occur. To date, most of the advancements have focused on new ways of writing the code that govern RTAP so that analysis of one or more data streams happens in a fraction of a second. Continued success in this area will depend on software, hardware, database and algorithm developers working together on complementary innovations.
For the security industry, RTAP improvements will focus on embedding highly complex analytics into the sensors and detectors that have traditionally gathered the data and transmitted it to other locations where processing and analysis occur. This applies to both physical and cyber sensors. For RTAP to be effective, the latency between capture and analysis must be eliminated or minimized. Putting analytic modeling capabilities right at the point of data capture within the sensor is the only way to accomplish this, and RTAP research is now focused on this element of the solution.
Aside from technological progress, the security profession must consider the changes it must make to incorporate RTAP into the protection of critical infrastructure. Based on experiences in other industries, the first step is merging security functionality with IT because RTAP is inherently an IT solution.
Next, is the willingness to break down stovepipes that may exist between various components of the security network – both cyber and physical – so that data feeds can be integrated and analyzed as part of a comprehensive solution. It is imperative to remember that infrastructure is now connected to the network where attacks occur in cyber space. Cyber and physical components are no longer separate.
Ultimately, the introduction of RTAP technology into the field of critical infrastructure security will come down to dollars and cents. The cost of early adoption will likely not be insignificant, but – as is always the case with security – the price will have to be weighed against the cascading impacts of a breach that results in the loss of a major facility or resource, such as a refinery, hydrocarbon pipeline or water supply.
Looking for a reprint of this article?
From high-res PDFs to custom plaques, order your copy today!